Unsupervised Image-to-Image Translation with Generative Prior
CVPR 2022
Paper
Abstract
Unsupervised image-to-image translation aims to learn the translation between two visual domains without paired data. Despite the recent progress in image translation models, it remains challenging to build mappings between complex domains with drastic visual discrepancies. In this work, we present a novel framework, Generative Prior-guided UNsupervised Image-to-image Translation (GP-UNIT), to improve the overall quality and applicability of the translation algorithm. Our key insight is to leverage the generative prior from pre-trained class-conditional GANs (e.g., BigGAN) to learn rich content correspondences across various domains. We propose a novel coarse-to-fine scheme: we first distill the generative prior to capture a robust coarse-level content representation that can link objects at an abstract semantic level, based on which fine-level content features are adaptively learned for more accurate multi-level content correspondences. Extensive experiments demonstrate the superiority of our versatile framework over state-of-the-art methods in robust, high-quality and diversified translations, even for challenging and distant domains.
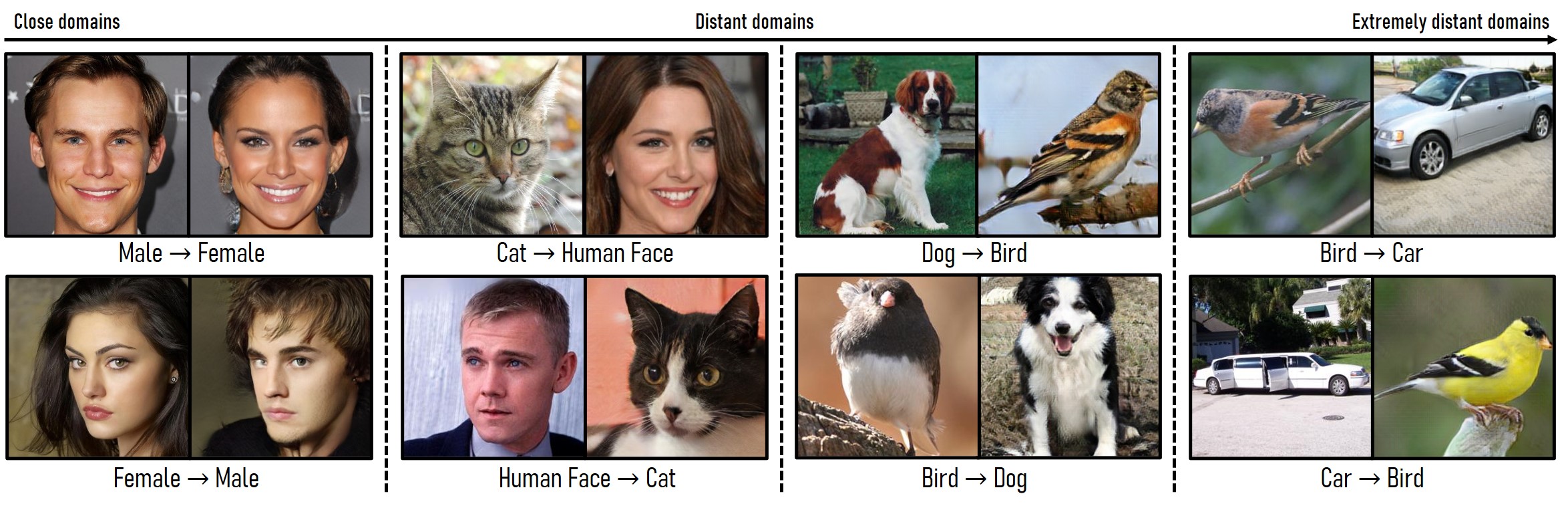
BigGAN
Generative Prior
Our framework is motivated by the following observation: objects generated by BigGAN, despite originating from different domains, share high content correspondences when generated from the same latent code. The interesting phenomenon suggests that there is an inherent content correspondence at a highly abstract semantic level regardless of the domain discrepancy in the BigGAN generative space. In particular, objects with the same latent code share either the same or very similar abstract representations in the first few layers, based on which domain-specific details are gradually added. GP-UNIT exploits this generative prior for building robust mappings and choose BigGAN for its rich cross-domain prior.
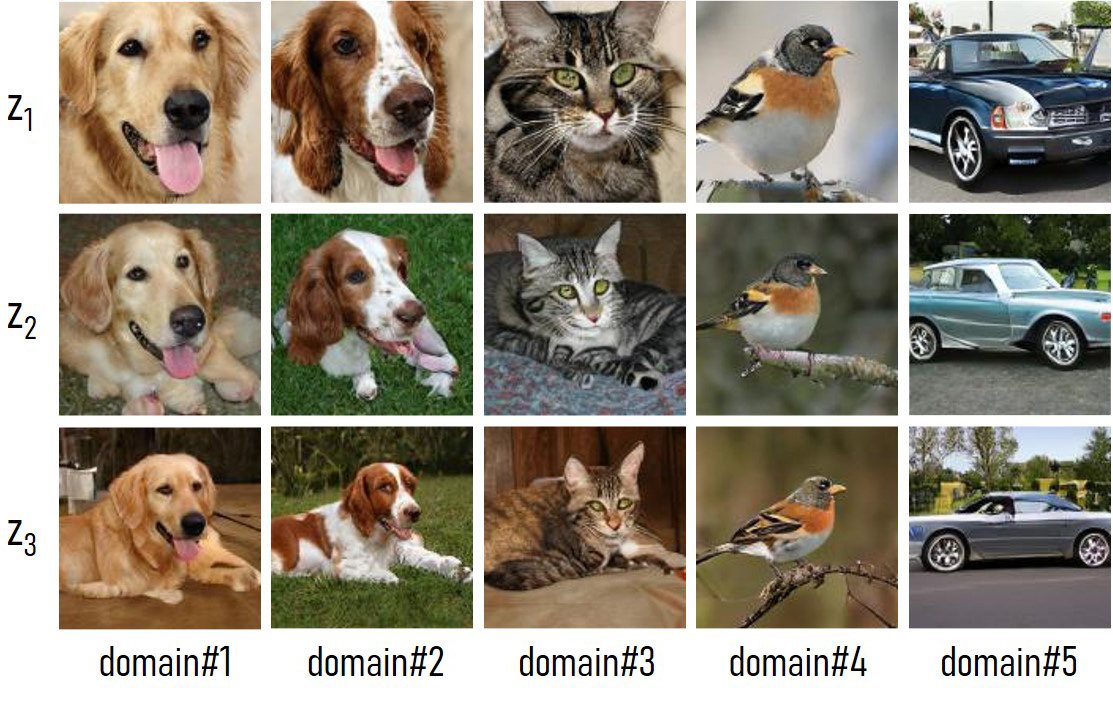
Generative space of BigGAN characterized by three latent codes (z1, z2, z3) across five domains. For each latent code, fine-grained correspondences can be observed between semantically related dogs and cats, such as the face features and body postures. For birds and vehicles, which are rather different, coarse-level correspondences in terms of orientation and layout can be observed.
The
GP-UNIT Framework
We decompose a translation task into coarse-to-fine stages: 1) generative prior distillation to learn robust cross-domain correspondences at a high semantic level and 2) adversarial image translation to build finer adaptable correspondences at multiple semantic levels. In the first stage, we train a content encoder to extract disentangled content representation by distilling the prior from the content-correlated data generated by BigGAN. In the second stage, we apply the pre-trained content encoder to the specific translation task, independent of the generative space of BigGAN, and propose a dynamic skip connection module to learn adaptable correspondences, so as to yield plausible and diverse translation results.
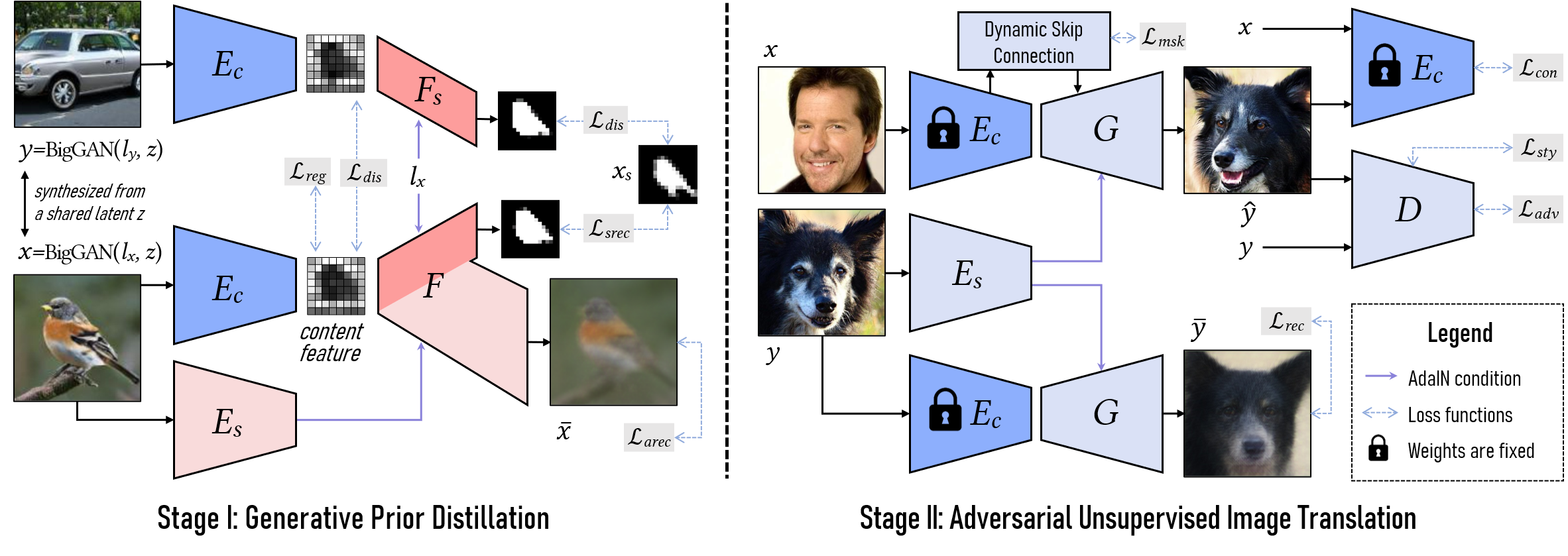
Translation
Between Various Domains
GP-UNIT expands the application scenarios of previous UNIT methods that mainly handles close domains. Our framework shows positive improvements over previous cycle-consistency-guided frameworks in: 1) capturing coarse-level correspondences across distant domains, beyond the ability of cycle-consistency guidance; 2) learning fine-level correspondences applicable to various tasks adaptively; and 3) only retaining essential content features in the coarse-to-fine stages, avoiding artifacts from the source domain.
Multi-Level
Content Correspondences
GP-UNIT is able to build valid multi-level content correspondences between different domains: coarse-level cross-domain content features at lv.0 are first built, based on which attentive masks at lv.1 and lv.2 adaptive to the task are gradually learned to pass the fine-level content features to the decoder.
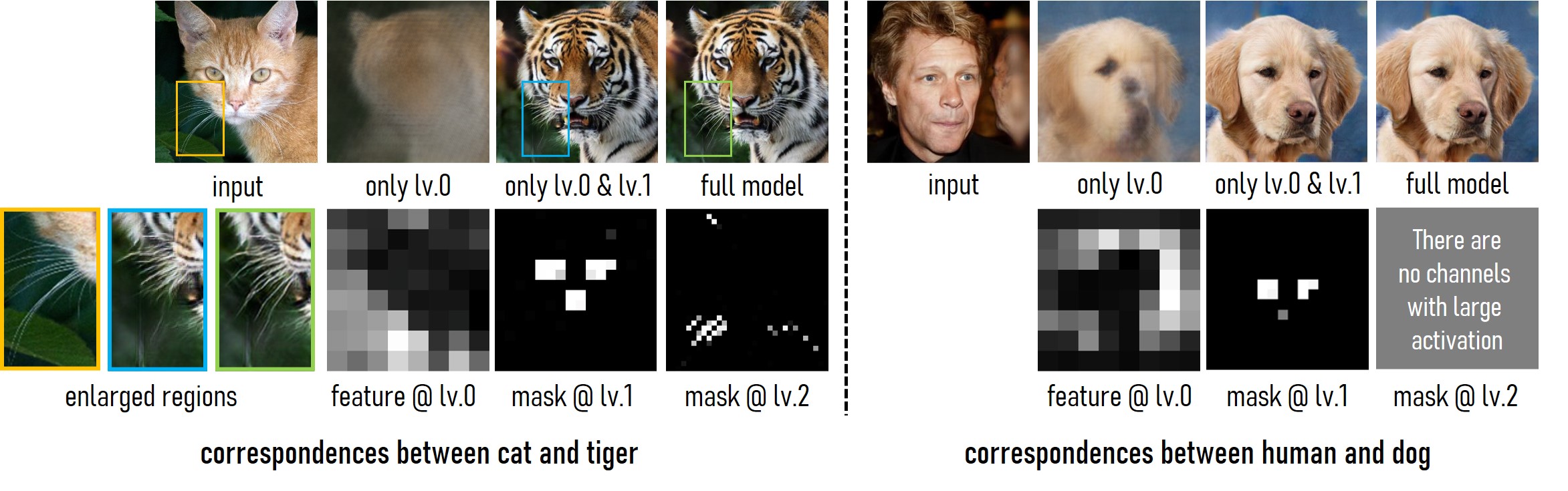
The most abstract content feature at lv.0 only gives layout cues. If we solely use it (by setting both masks at lv.1 and lv.2 to all-zero tensors), the resulting tiger and dog faces have no details. Meanwhile, correspondences at lv.1 focus on mid-level details like the nose and eyes of cat face, and eyes of human face, which is enough to generate a realistic result with the coarse-level features. Finally, correspondences at lv.2 pays attention to subtle details like the cat whiskers for close domains. Therefore, our full multi-level content features enable us to simulate the extremely fine-level long whiskers in the input. As expected, such kind of fine-level correspondences are not found between more distant human and dog faces, preventing the unwanted appearance influence from the source domain. Such reasonable and adaptable semantic attentions are learned merely via the generation prior, without any explicit correspondence supervision.
Experimental
Results
Comparison with state-of-the-art methods
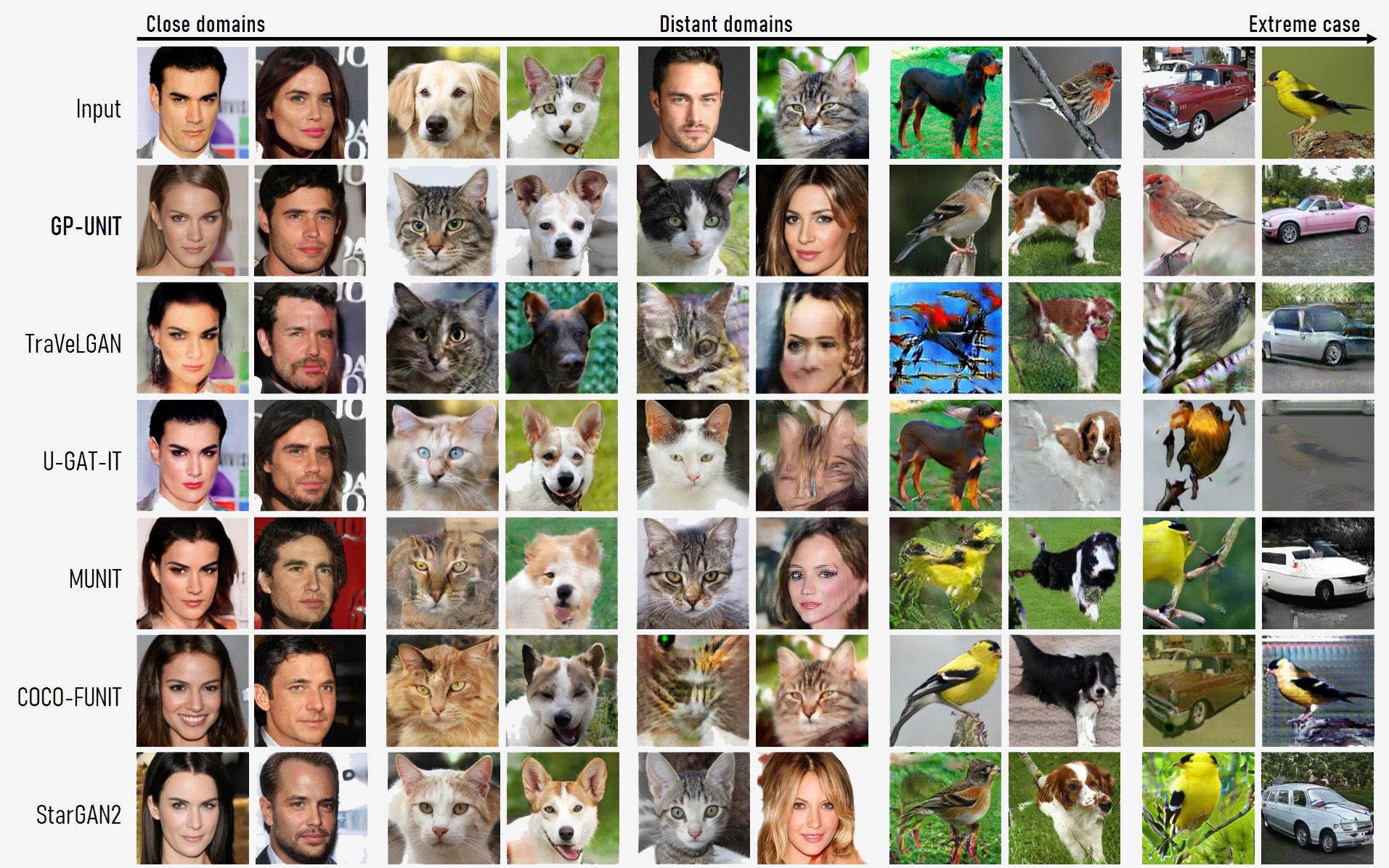
We perform visual comparison to six state-of-the-art methods: TraVeLGAN, U-GAT-IT, MUNIT, COCO-FUNIT and StarGAN2. Cycle-consistency-guided U-GAT-IT, MUNIT and StarGAN2 rely on the low-level cues of the input image for bi-directional reconstruction, which leads to some undesired artifacts, such as the distorted cat face region that corresponds to the dog ears, and the ghosting dog legs in the generated bird images. Meanwhile, TraVeLGAN and COCO-FUNIT fail to build proper content correspondences for Human Face ↔ Cat and Bird ↔ Car. By comparison, our method is comparable to the above methods on Male ↔ Female task and show consistent superiority on other challenging tasks.
Performance on domains beyond BigGAN
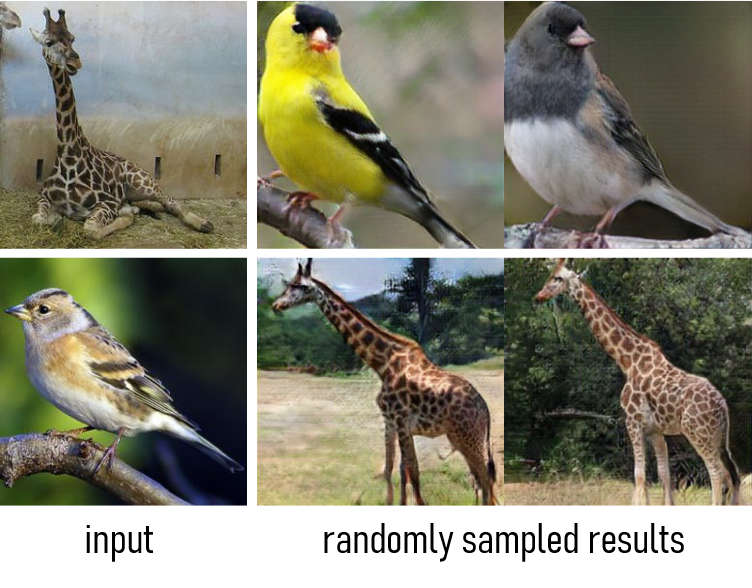
Giraffe ↔ Bird Translation
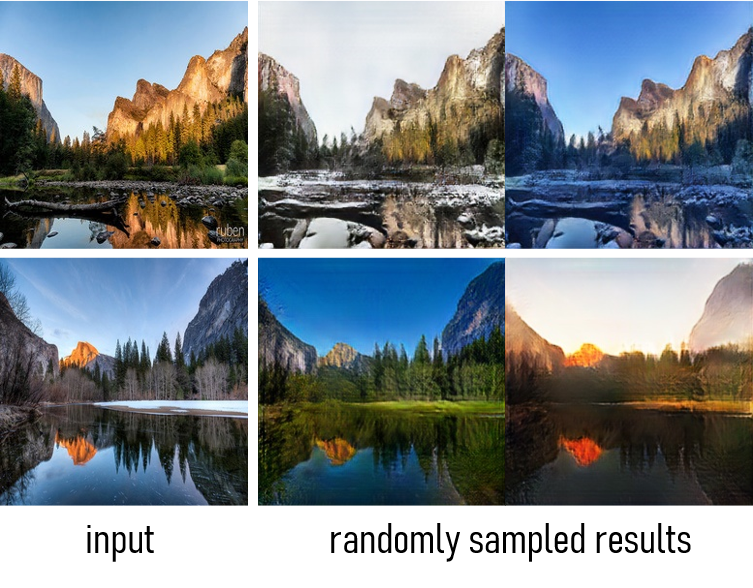
Summer ↔ Winter Translation
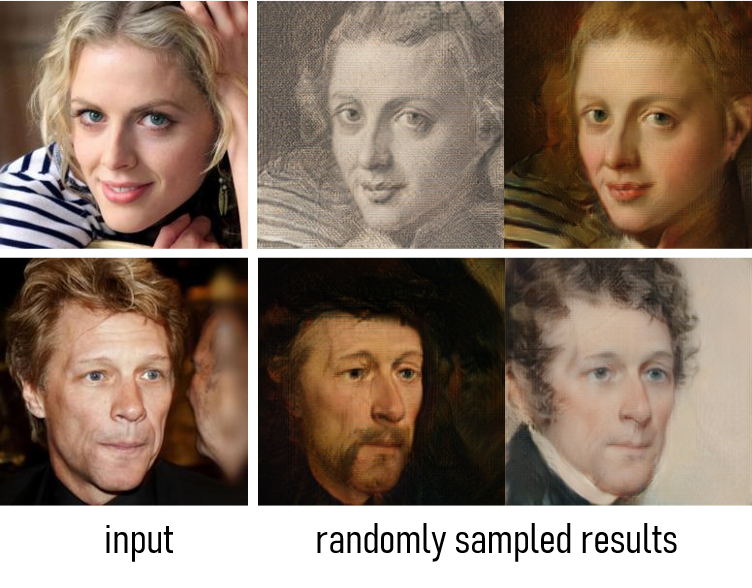
Real Face → Art Translation
Paper
Citation
@InProceedings{yang2022Unsupervised,
author = {Yang, Shuai and Jiang, Liming and Liu, Ziwei and and Loy, Chen Change},
title = {Unsupervised Image-to-Image Translation with Generative Prior},
booktitle = {Proceedings of the IEEE/CVF Conference on Computer Vision and Pattern Recognition},
year = {2022}
}
Related
Projects
-
Exploiting Deep Generative Prior for Versatile Image Restoration and Manipulation
X. Pan, X. Zhan, B. Dai, D. Lin, C. C. Loy, P. Luo
in Proceedings of European Conference on Computer Vision, 2020 (ECCV, Oral)
[PDF] [arXiv] [Project Page] -
TSIT: A Simple and Versatile Framework for Image-to-Image Translation
L. Jiang, C. Zhang, M. Huang, C. Liu, J. Shi, C. C. Loy
in Proceedings of European Conference on Computer Vision, 2020 (ECCV, Spotlight)
[PDF] [arXiv] [Project Page] -
TransGaGa: Geometry-Aware Unsupervised Image-to-Image Translation
W. Wu, K. Cao, C. Li, C. Qian, C. C. Loy
in Proceedings of IEEE Conference on Computer Vision and Pattern Recognition, 2019 (CVPR)
[PDF] [arXiv] [Project Page]
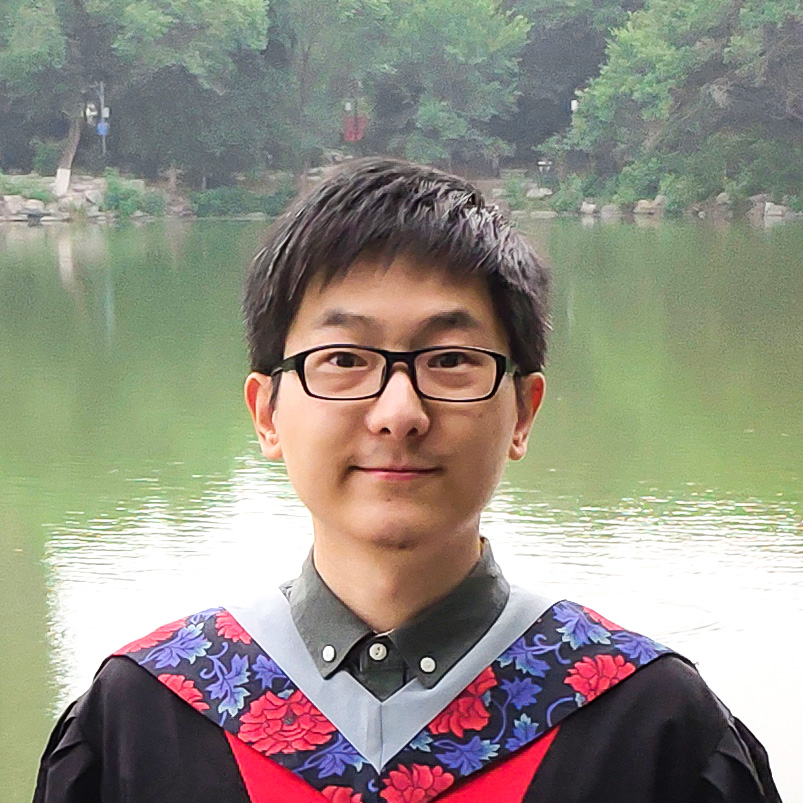