Open-Vocabulary SAM: Segment and Recognize Twenty-thousand Classes Interactively
arXiv pre-print, 2024
Paper
Abstract
The CLIP and Segment Anything Model (SAM) are remarkable vision foundation models (VFMs). SAM excels in segmentation tasks across diverse domains, while CLIP is renowned for its zero-shot recognition capabilities. This paper presents an in-depth exploration of integrating these two models into a unified framework. Specifically, we introduce the Open-Vocabulary SAM, a SAM-inspired model designed for simultaneous interactive segmentation and recognition, leveraging two unique knowledge transfer modules: SAM2CLIP and CLIP2SAM. The former adapts SAM's knowledge into the CLIP via distillation and learnable transformer adapters, while the latter transfers CLIP knowledge into SAM, enhancing its recognition capabilities. Extensive experiments on various datasets and detectors show the effectiveness of Open-Vocabulary SAM in both segmentation and recognition tasks, significantly outperforming the naive baselines of simply combining SAM and CLIP. Furthermore, aided with image classification data training, our method can segment and recognize approximately 22,000 classes.
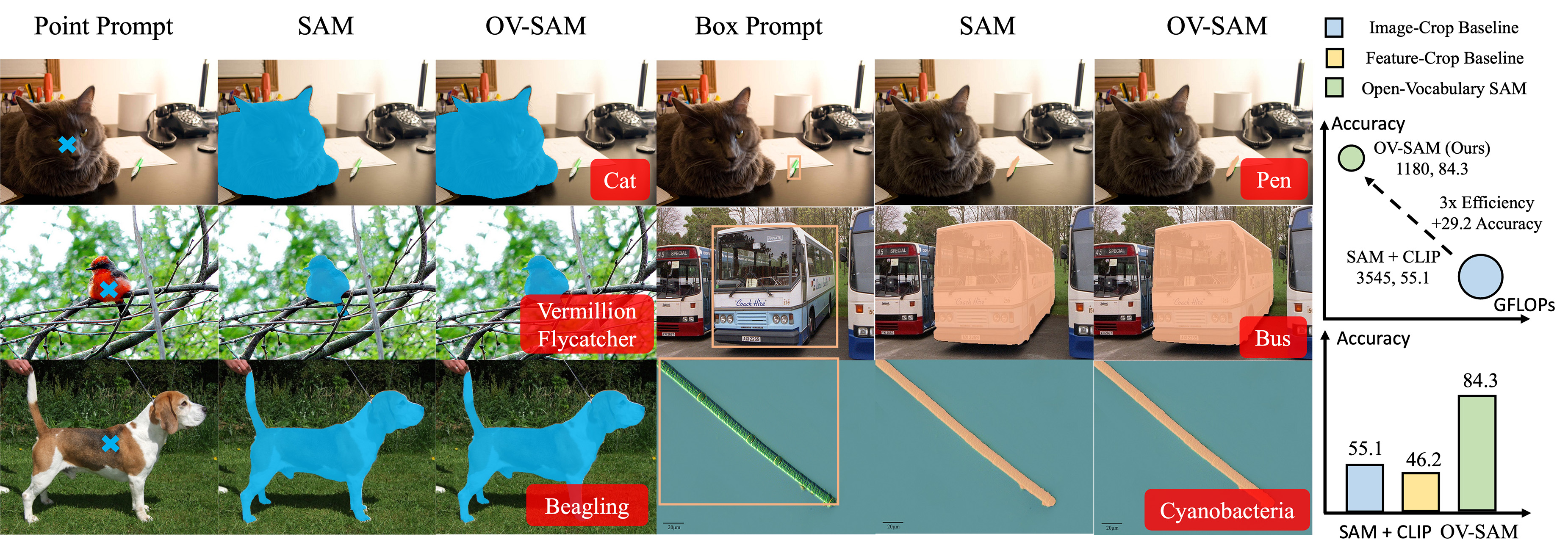
Open-Vocabulary SAM extends SAM's segmentation capabilities with CLIP-like real-world recognition, while significantly reducing computational costs. It outperforms combined SAM and CLIP methods in object recognition on the COCO open vocabulary benchmark.
THE FRAMEWORK
Open-Vocabulary SAM
Open-Vocabulary SAM fuses the knowledge from both SAM and CLIP to a unified architecture. This unified architecture is made possible via the SAM2CLIP, which transfers knowledge of SAM to CLIP with distillation, and CLIP2SAM, which employs CLIP knowledge and combines the SAM mask decoder for recognition. For training, the SAM encoder is adopted as a teacher network, while SAM2CLIP plays the role of a student network and aligns the knowledge of SAM into CLIP. The CLIP2SAM transfers the CLIP knowledge to the SAM decoder and performs joint segmentation and classification for close-set and open vocabulary settings.
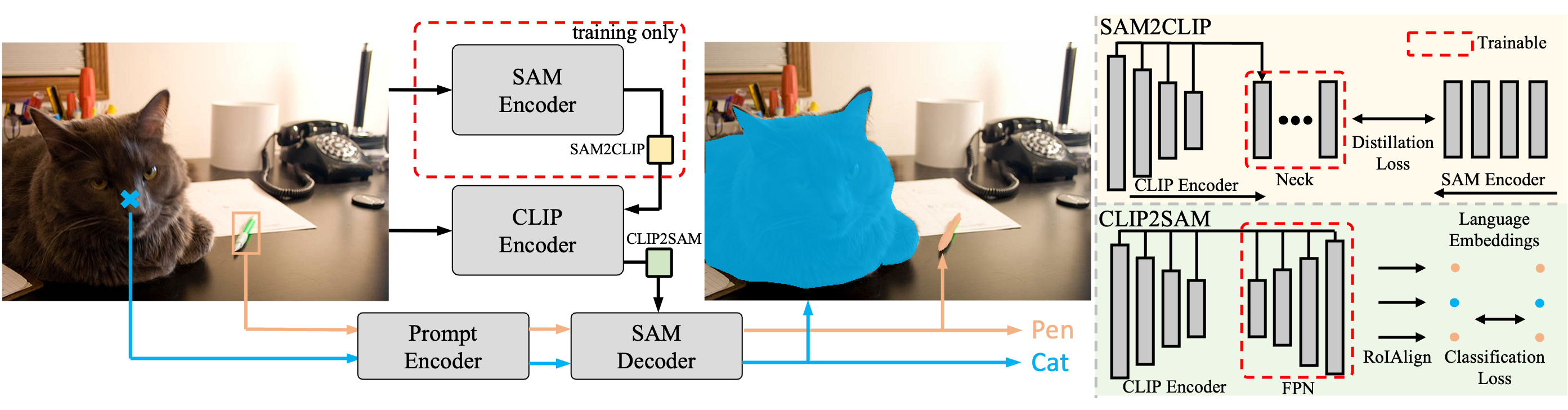
interactive
Demo
We provide a web demo for interactive segmentation and recognition. You can upload your own image and draw a bounding box to segment and recognize the object. Please check our web demo here.
Click to change examples ▼
Experimental
Results
Comparison with combined SAM and CLIP baselines
We report the results on COCO and LVIS Open-Vocabulary benchmarks of Open-Vocabulary SAM and the combined baselines using ground truth boxes. IoUb and IoUn refer to the average IoU for each mask of base classes and novel classes, respectively.
Method | COCO | LVIS | FLOPs (G) | #Params (M) | ||||
---|---|---|---|---|---|---|---|---|
IoUb | IoUn | Acc | IoUb | IoUn | Acc | |||
Image-Crop baseline | 78.1 | 81.4 | 46.2 | 78.3 | 81.6 | 9.6 | 3,748 | 808 |
Feature-Crop baseline | 78.1 | 81.4 | 55.1 | 78.3 | 81.6 | 26.5 | 3,545 | 808 |
Image-Crop baseline + Adapter1 | 79.6 | 82.1 | 62.0 | 80.1 | 82.0 | 32.1 | 3,748 | 808 |
Feature-Crop baseline + Adapter1 | 79.6 | 82.1 | 70.9 | 80.1 | 82.0 | 48.2 | 3,545 | 808 |
Feature-Crop baseline + Adapter2 | 78.5 | 80.9 | 75.3 | 79.5 | 81.8 | 29.6 | 3,590 | 828 |
Feature-Crop baseline + Adapter3 | 76.1 | 79.3 | 63.1 | 76.1 | 79.2 | 21.0 | 3,580 | 812 |
Open-Vocabulary SAM (ours) | 81.5 | 84.0 | 84.3 | 80.4 | 83.1 | 66.6 | 1,180 | 304 |
Visualization Results
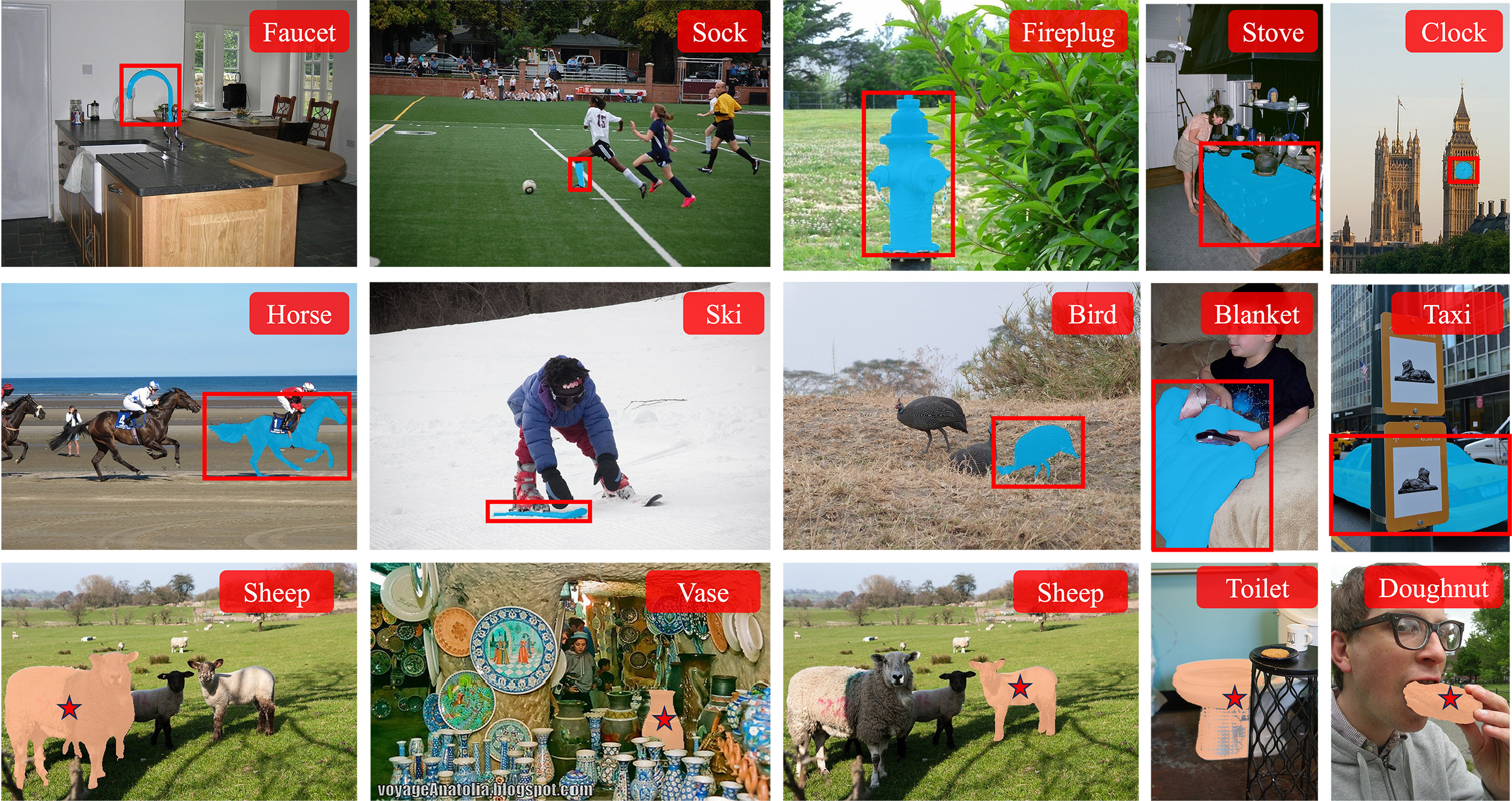
Paper
Citation
@article{yuan2024ovsam,
title={Open-Vocabulary SAM: Segment and Recognize Twenty-thousand Classes Interactively},
author={Yuan, Haobo and Li, Xiangtai and Zhou, Chong and Li, Yining and Chen, Kai and Loy, Chen Change},
journal={arXiv preprint},
year={2024}
}
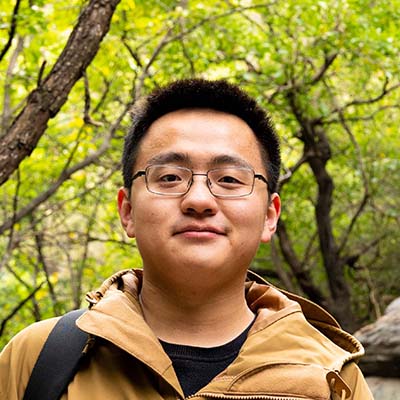