VToonify: Controllable High-Resolution Portrait Video Style Transfer
ACM TOG (SIGGRAPH Asia), 2022
Paper
Abstract
Generating high-quality artistic portrait videos is an important and desirable task in computer graphics and vision. Although a series of successful portrait image toonification models built upon the powerful StyleGAN have been proposed, these image-oriented methods have obvious limitations when applied to videos, such as the fixed frame size, the requirement of face alignment, missing non-facial details and temporal inconsistency. In this work, we investigate the challenging controllable high-resolution portrait video style transfer by introducing a novel VToonify framework. Specifically, VToonify leverages the mid- and high-resolution layers of StyleGAN to render high-quality artistic portraits based on the multi-scale content features extracted by an encoder to better preserve the frame details. The resulting fully convolutional architecture accepts non-aligned faces in videos of variable size as input, contributing to complete face regions with natural motions in the output. Our framework is compatible with existing StyleGAN-based image toonification models to extend them to video toonification, and inherits appealing features of these models for flexible style control on color and intensity. This work presents two instantiations of VToonify built upon Toonify and DualStyleGAN for collection-based and exemplar-based portrait video style transfer, respectively. Extensive experimental results demonstrate the effectiveness of our proposed VToonify framework over existing methods in generating high-quality and temporally-coherent artistic portrait videos with flexible style controls.
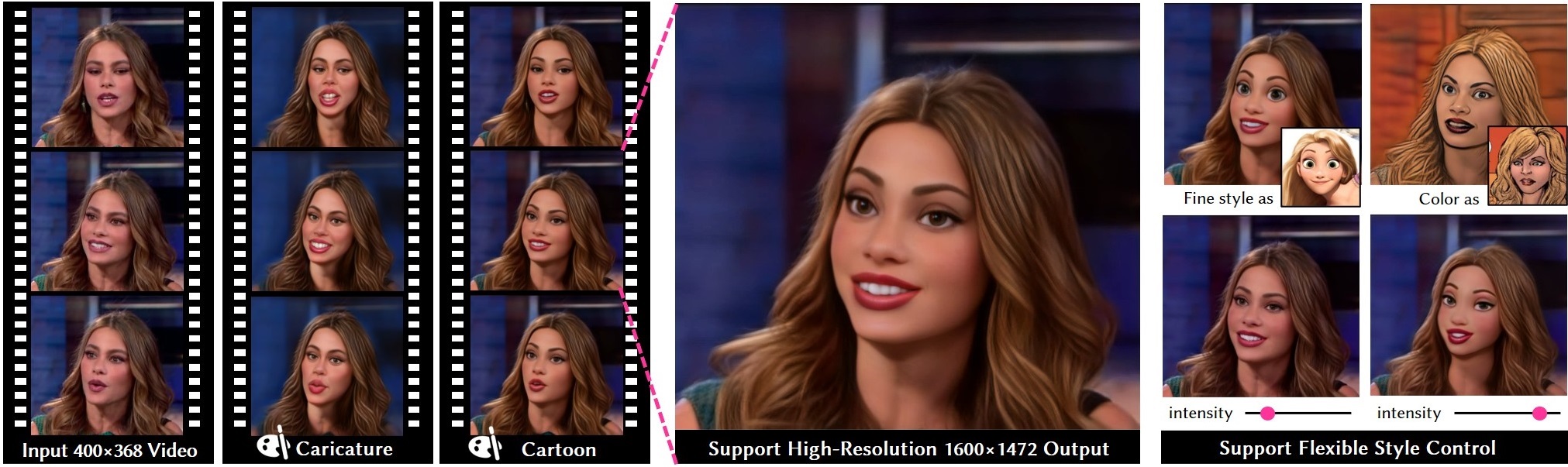
The
VToonify Framework
VToonify combines the merits of the image translation framework and the StyleGAN-based framework to achieve controllable high-resolution portrait video style transfer. (a) Image translation framework uses fully convolutional networks to support variable input size. However, training from scratch renders it difficult for high-resolution and controllable style transfer. (b) StyleGAN-based framework leverages the pre-trained StyleGAN model for high-resolution and controllable style transfer, but is limited to fixed image size and detail losses. (c) Our hybrid framework adapts StyleGAN by removing its fixed-sized input feature and low-resolution layers to construct a fully convolutional encoder-generator architecture akin to that in the image translation framework. We train an encoder to extract multi-scale content features of the input frame as the additional content condition to the generator to preserve frame details. By incorporating the StyleGAN model into the generator to distill both its data and model, VToonify inherits the style control flexibility of StyleGAN.
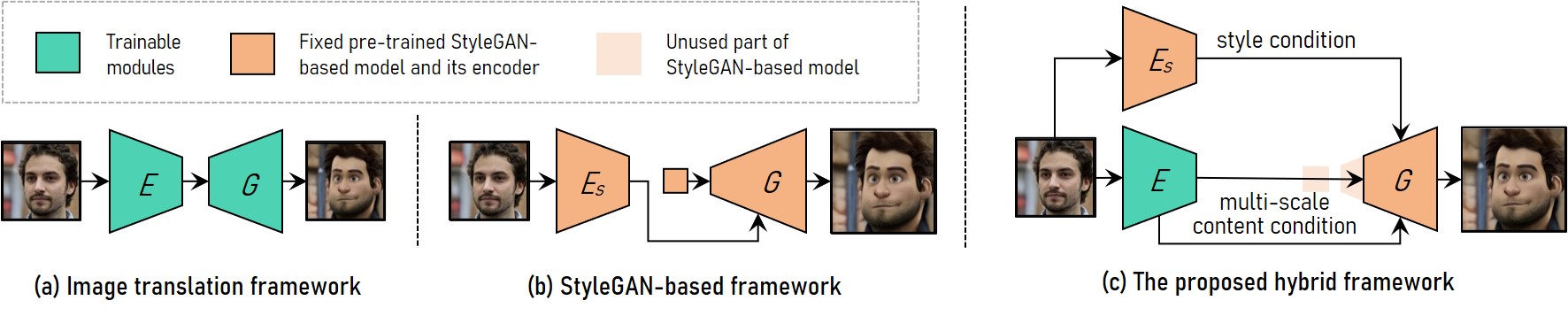
Toonification with
Flexible Style Control
Our framework is compatible with the existing StyleGAN-based image toonification models to extend them to video toonification, and inherits their appealing features for flexible style control. Taking the DualStyleGAN model as the StyleGAN backbone, our VToonify provides: 1) exemplar-based structure style transfer, 2) adjustment of style degree 3) exemplar-based color style transfer.
Style control
Experimental
Results
Comparison with state-of-the-art face style transfer methods
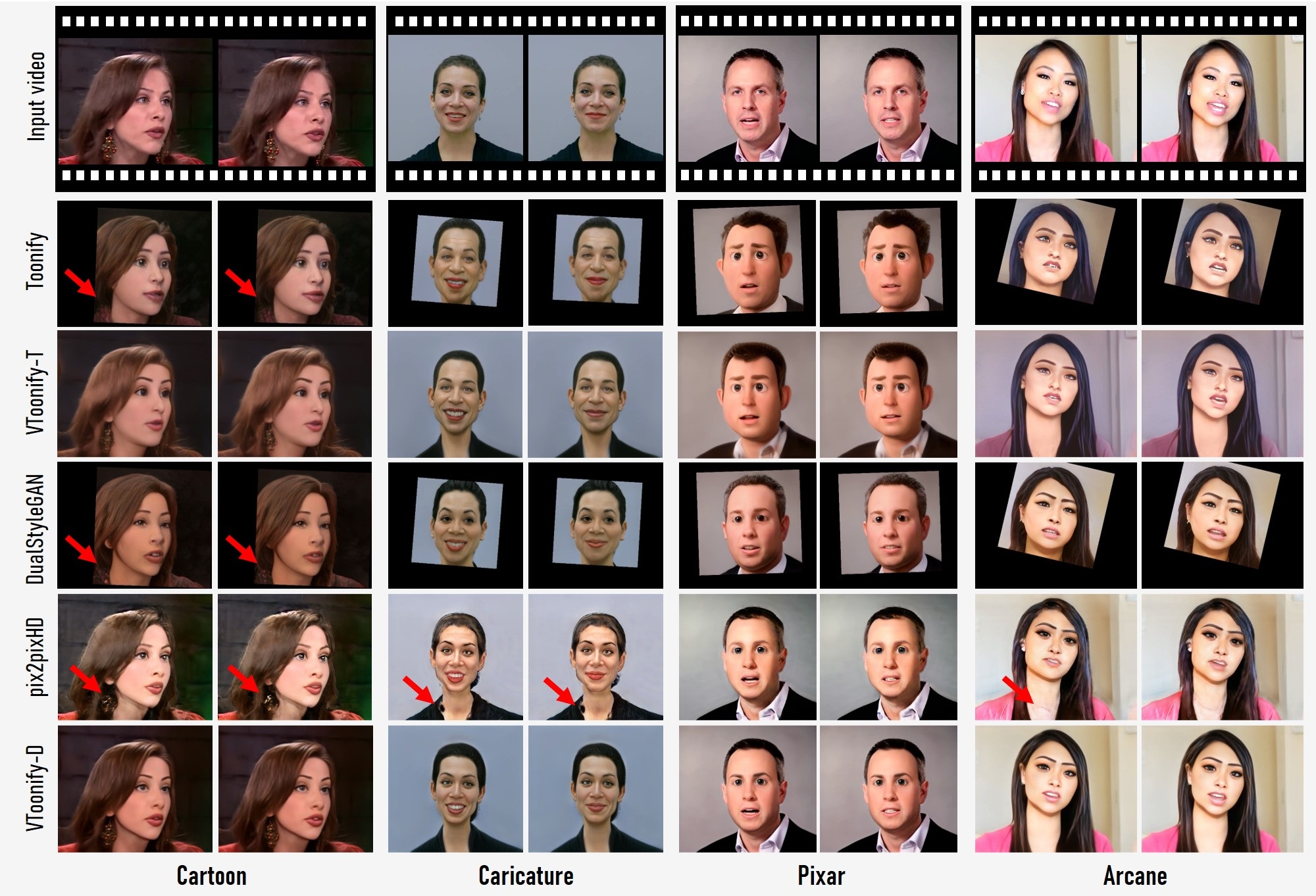
We perform visual comparison to our two backbones Toonify, DualStyleGAN and the high-resolution image-to-image translation baseline Pix2pixHD for StyleGAN distillation. VToonify-T and VToonify-D surpass their corresponding backbones Toonify and DualStyleGAN in stylizing the complete video, while maintaining the same high quality and visual features as the backbones for each single frame. For example, VToonify-T follows Toonify to impose a strong style effect like violet hair in the Arcane style. VToonify-D, on the other hand, is better at preserving the facial features. Compared with VToonify-D, Pix2pixHD suffers from flickers and artifacts.
Face toonification on more styles
Paper
Citation
@article{yang2022vtoonify,
title = {VToonify: Controllable High-Resolution Portrait Video Style Transfer},
author = {Yang, Shuai and Jiang, Liming and Liu, Ziwei and and Loy, Chen Change},
journal = {ACM Transactions on Graphics (TOG)},
volume = {41},
number = {6},
articleno = {203},
pages = {1--15},
year = {2022},
publisher = {ACM New York, NY, USA},
doi={10.1145/3550454.3555437},
}
Related
Projects
-
Pastiche Master: Exemplar-Based High-Resolution Portrait Style Transfer
S. Yang, L. Jiang, Z. Liu, C. C. Loy
in Proceedings of IEEE Conference on Computer Vision and Pattern Recognition, 2022 (CVPR)
[PDF] [arXiv] [Project Page] -
Deep Plastic Surgery: Robust and Controllable Image Editing with Human-Drawn Sketches
S. Yang, Z. Wang, J. Liu, Z. Guo
in Proceedings of European Conference on Computer Vision, 2020 (ECCV)
[PDF] [arXiv] [Project Page] -
Talk-to-Edit: Fine-Grained Facial Editing via Dialog
Y. Jiang, Z. Huang, X. Pan, C. C. Loy, Z. Liu
in Proceedings of IEEE/CVF International Conference on Computer Vision, 2021 (ICCV)
[PDF] [arXiv] [Project Page]
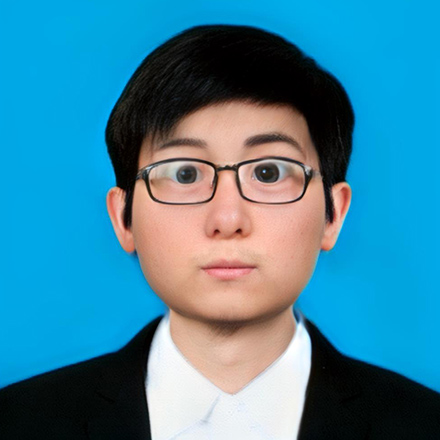