DeformToon3D: Deformable 3D Toonification from Neural Radiance Fields
ICCV 2023
Paper
Abstract
In this paper, we address the challenging problem of 3D toonification, which involves transferring the style of an artistic domain onto a target 3D face with stylized geometry and texture. Although fine-tuning a pre-trained 3D GAN on the artistic domain can produce reasonable performance, this strategy has limitations in the 3D domain. In particular, fine-tuning can deteriorate the original GAN latent space, which affects subsequent semantic editing, and requires independent optimization and storage for each new style, limiting flexibility and efficient deployment. To overcome these challenges, we propose DeformToon3D, an effective toonification framework tailored for hierarchical 3D GAN. Our approach decomposes 3D toonification into subproblems of geometry and texture stylization to better preserve the original latent space. Specifically, we devise a novel StyleField that predicts conditional 3D deformation to align a real-space NeRF to the style space for geometry stylization. Thanks to the StyleField formulation, which already handles geometry stylization well, texture stylization can be achieved conveniently via adaptive style mixing that injects information of the artistic domain into the decoder of the pre-trained 3D GAN. Due to the unique design, our method enables flexible style degree control and shape-texture-specific style swap. Furthermore, we achieve efficient training without any real-world 2D-3D training pairs but proxy samples synthesized from off-the-shelf 2D toonification models.
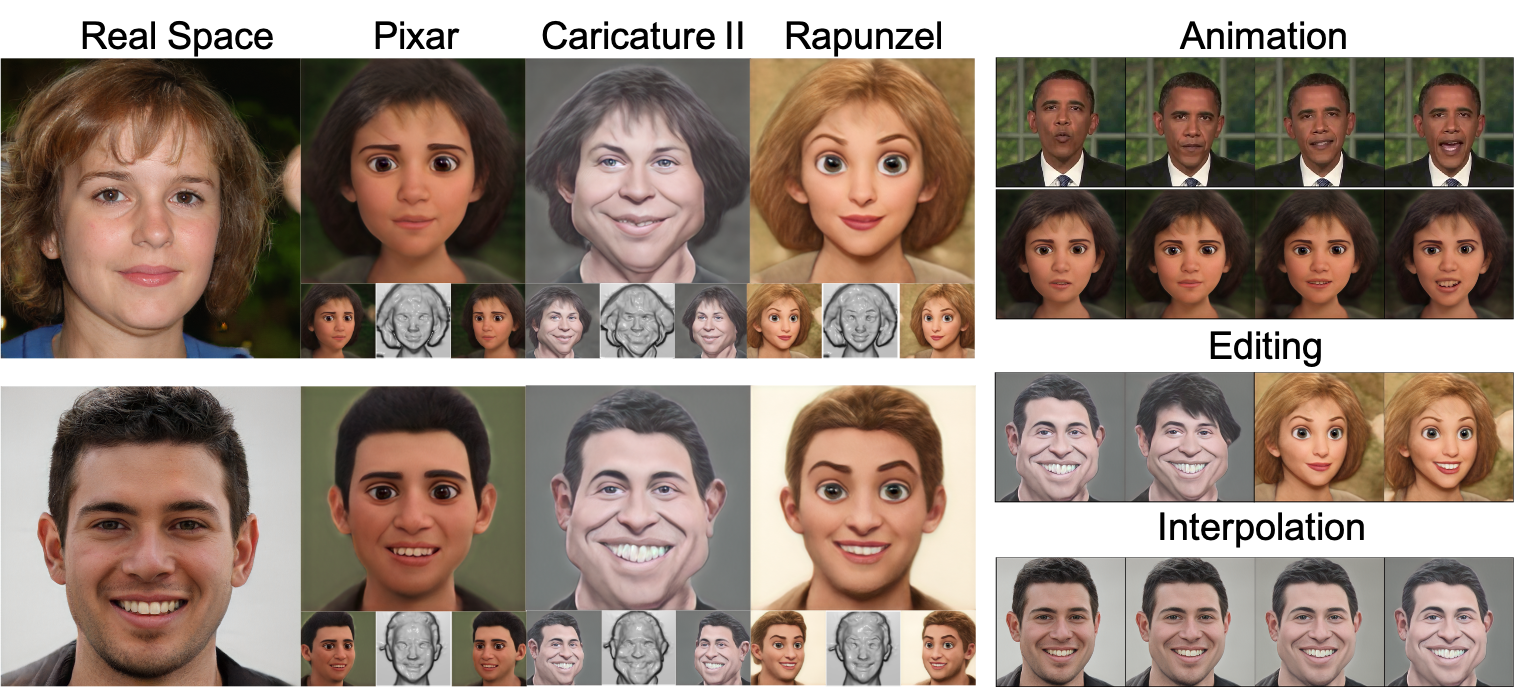
DeformToon3D
Framework
Given sampled instance code wR and style code wS as conditions, DeformToon3D first deforms a point from the style space NS to the real space NR, which achieves geometry toonification without modifying the pre-traine G0. Afterwards, we leverage adaptive style mixing with weight w to inject the texture information of the target domain into the pre-trained G1 for texture toonification. The original 3D-aware real face generation is shown in "GREEN" line, and the DeformToon3D domain adaptation generation is shown in "RED" line. Both pre-trained generators G0 and G1 are kept frozen.
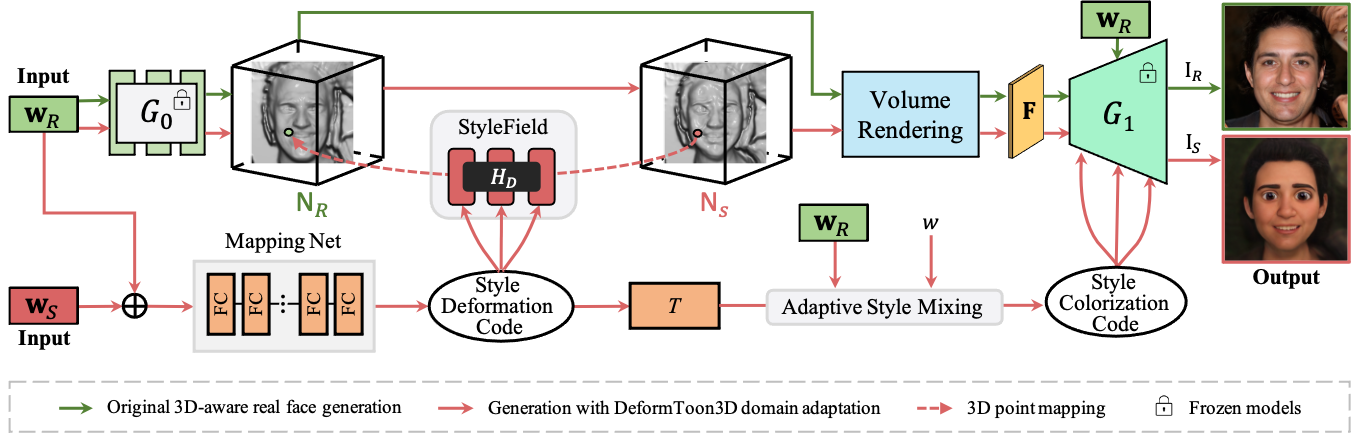
Qualitative
Results
The unique design of DeformToon3D avails several notable features. Unlike conventional 3D toonification approaches that fine-tune the whole 3D GAN generator to a specific style, our framework is capable of achieving high-quality geometry and texture toonification with a range of styles housed in a single model. Due to the preservation of 3D GAN latent space, it could leverage existing GAN editing tools for toonification editing, and also support animation of the 3D toonification. Furthermore, thanks to the disentanglement of geometry and texture, it enables flexible geometry, texture, and full interpolation.
Paper
Citation
@inproceedings{zhang2023deformtoon3d,
title = {DeformToon3D: Deformable 3D Toonification from Neural Radiance Fields},
author = {Junzhe Zhang, Yushi Lan, Shuai Yang, Fangzhou Hong, Quan Wang, Chai Kiat Yeo, Ziwei Liu, Chen Change Loy},
booktitle = {ICCV},
year = {2023}}
Related
Projects
-
E3DGE: Self-supervised Geometry-Aware Encoder for Style-based 3D GAN Inversion
Y. Lan, X. Meng, S. Yang, C. C. Loy, B. Dai
in Proceedings of IEEE Conference on Computer Vision and Pattern Recognition, 2023 (CVPR)
[Paper] [Project Page] -
VToonify: Controllable High-Resolution Portrait Video Style Transfer
S. Yang, L. Jiang, Z. Liu, C. C. Loy
ACM Transactions on Graphics, 2022 (SIGGRAPH Asia 2022)
[PDF] [arXiv] [Project Page] -
Pastiche Master: Exemplar-Based High-Resolution Portrait Style Transfer
S. Yang, L. Jiang, Z. Liu, C. C. Loy
in Proceedings of IEEE Conference on Computer Vision and Pattern Recognition, 2022 (CVPR)
[PDF] [arXiv] [Project Page]
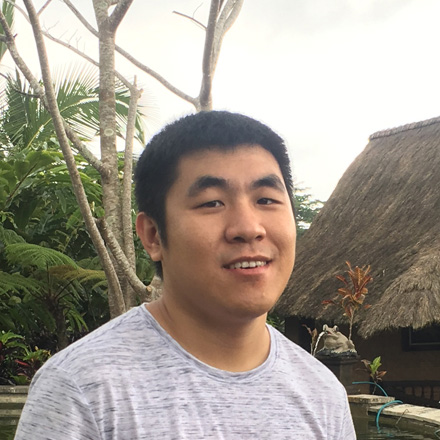