StyleInV: A Temporal Style Modulated Inversion Network for Unconditional Video Generation
ICCV, 2023
Paper
Abstract
Unconditional video generation is a challenging task that involves synthesizing high-quality videos that are both coherent and of extended duration. To address this challenge, researchers have used pretrained StyleGAN image generators for high-quality frame synthesis and focused on motion generator design. The motion generator is trained in an autoregressive manner using heavy 3D convolutional discriminators to ensure motion coherence during video generation. In this paper, we introduce a novel motion generator design that uses a learning-based inversion network for GAN. The encoder in our method captures rich and smooth priors from encoding images to latents, and given the latent of an initially generated frame as guidance, our method can generate smooth future latent by modulating the inversion encoder temporally. Our method enjoys the advantage of sparse training and naturally constrains the generation space of our motion generator with the inversion network guided by the initial frame, eliminating the need for heavy discriminators. Moreover, our method supports style transfer with simple fine-tuning when the encoder is paired with a pretrained StyleGAN generator. Extensive experiments conducted on various benchmarks demonstrate the superiority of our method in generating long and high-resolution videos with decent single-frame quality and temporal consistency.
Methodology
Model Framework
We present an effective framework for non-autoregressive motion generation that is capable of generating long and high-resolution videos. Our approach leverages learning-based Generative Adversarial Network (GAN) inversion, which learns the inverse mapping of GANs via an inversion network that consists of an encoder and a decoder. To generate long and coherent videos, we exploit the unique characteristic of the inversion encoder, which captures a rich and smooth manifold between the mapping of images and latent. To generate a sequence of smooth motion latents, we just need to provide the initial latent code and modulate the inversion encoder with temporal style codes, which are encodings of timestamps with randomness. The motion latents can then be mapped by a StyleGAN decoder to generate a video.
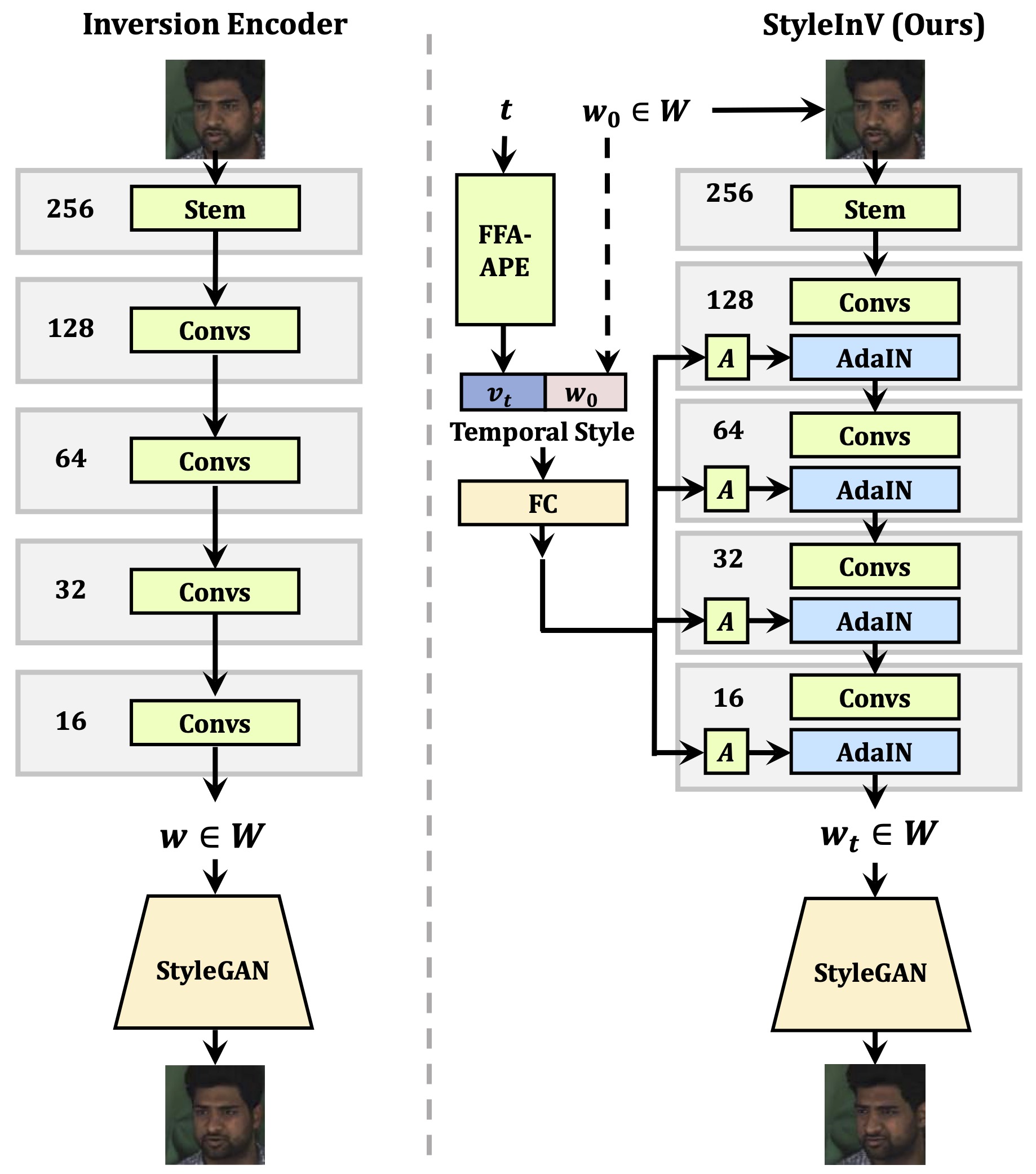
Methodology
Finetuning-based Style Transfer
Our 'inversion encoder+decoder' framework can naturally take a pretrained StyleGAN model as the generator. And such a configuration allows the generator to be fine-tuned for different styles, and yet still able to use the motion generator for generating new video with styles. The capability is not possible with existing non-autoregressive video generation methods because they cannot be finetuned under an image GAN training scheme. This feature module is plug-and-play and does not introduce any inference latency.
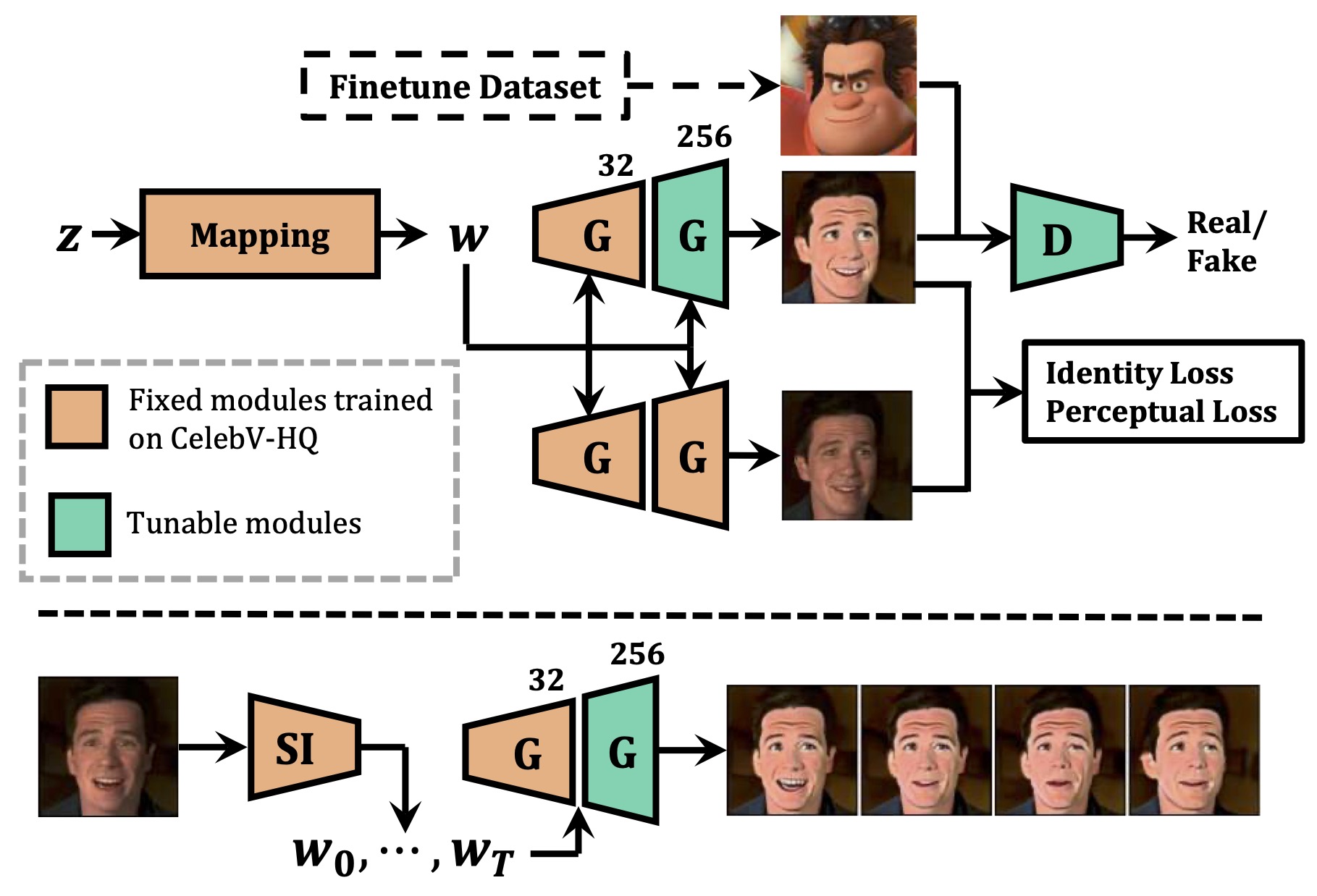
Qualitative
Comparison
Compared to existing methods, our method demonstrates stable results on all four datasets, particularly with superior identity preservation on human-face video and long-term generation quality on TaiChi. Although our method outperforms existing methods in terms of content quality, continuity, and quantitative results, the motion semantics of our generated videos on SkyTimelapse are inferior to those on other datasets. This could be one of the limitations of our work and an area for future improvement.
More
Applications
Finetuning-based style transfer
From left to right: Raw generated content, Cartoon style, Arcane style, and Metface style.
We train the parent model (motion generator and StyleGAN) on CelebV-HQ, as its rich identity makes it more suitable for transfer learning. To perform style transfer, we fine-tune the StyleGAN on the Cartoon, MetFace, and Arcane datasets. Our method achieves satisfactory results in terms of smooth video style transfer with well-aligned face structure, identity, and expression, demonstrating its desirable properties and potential for various applications.
Initial-frame conditioned generation
From left to right: In-the-wild image, pSp inversion, Raw generated video, Style-transfer result.
Our network supports generating a series of content given a real-world image as the initial frame. We first inverse the image into the StyleGAN2 latent space with a pSp encoder, which is trained to initialize the weights of StyleInV. We treat it as the 512-dimensional initial frame latent, then use it to generate a video with our StyleInV. The generated latent sequence can be also applied to a finetuned image generator to synthesize a style-transferred animation video.
Paper
Citation
@InProceedings{wang2023styleinv,
title = {{StyleInV}: A Temporal Style Modulated Inversion Network for Unconditional Video Generation},
author = {Wang, Yuhan and Jiang, Liming and Loy, Chen Change},
booktitle = {ICCV},
year = {2023},
}
Related
Projects
-
DeeperForensics-1.0: A Large-Scale Dataset for Real-World Face Forgery Detection
L. Jiang, R. Li, W. Wu, C. Qian, C. C. Loy
in Proceedings of IEEE Conference on Computer Vision and Pattern Recognition, 2020 (CVPR)
[PDF] [arXiv] [Supplementary Material] [Project Page] -
CelebV-HQ: A Large-Scale Video Facial Attributes Dataset
H. Zhu, W. Wu, W. Zhu, L. Jiang, S. Tang, L. Zhang, Z. Liu, C. C. Loy
European Conference on Computer Vision, 2022 (ECCV)
[PDF] [arXiv] [Supplementary Material] [Project Page] -
VToonify: Controllable High-Resolution Portrait Video Style Transfer
S. Yang, L. Jiang, Z. Liu, C. C. Loy
ACM Transactions on Graphics, 2022 (SIGGRAPH Asia - TOG)
[PDF] [arXiv] [Project Page]
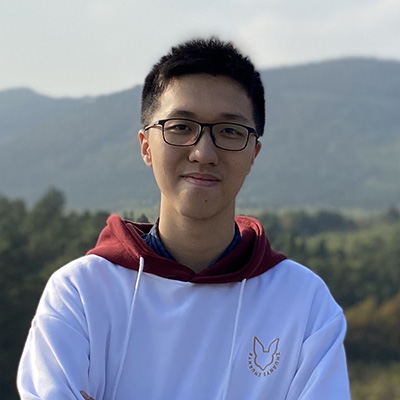