FRESCO: Spatial-Temporal Correspondence for Zero-Shot Video Translation
CVPR 2024
Paper
Abstract
The remarkable efficacy of text-to-image diffusion models has motivated extensive exploration of their potential application in video domains. Zero-shot methods seek to extend image diffusion models to videos without necessitating model training. Recent methods mainly focus on incorporating inter-frame correspondence into attention mechanisms. However, the soft constraint imposed on determining where to attend to valid features can sometimes be insufficient, resulting in temporal inconsistency. In this paper, we introduce FRESCO, intra-frame correspondence alongside inter-frame correspondence to establish a more robust spatial-temporal constraint. This enhancement ensures a more consistent transformation of semantically similar content across frames. Beyond mere attention guidance, our approach involves an explicit update of features to achieve high spatial-temporal consistency with the input video, significantly improving the visual coherence of the resulting translated videos.
The
FRamE Spatial-temporal COrrespondence
We present FRamE Spatial-temporal COrrespondence (FRESCO). While previous methods primarily focus on constraining inter-frame temporal correspondence, we believe that preserving intra-frame spatial correspondence is equally crucial. Our approach ensures that semantically similar content is manipulated cohesively, maintaining its similarity post-translation. This strategy enhances the consistency of the optical flow during manipulation to prevent inconsistent guidance. In addition, for regions where optical flow is not available, the spatial correspondence within the original frame can serve as a regulatory mechanism.
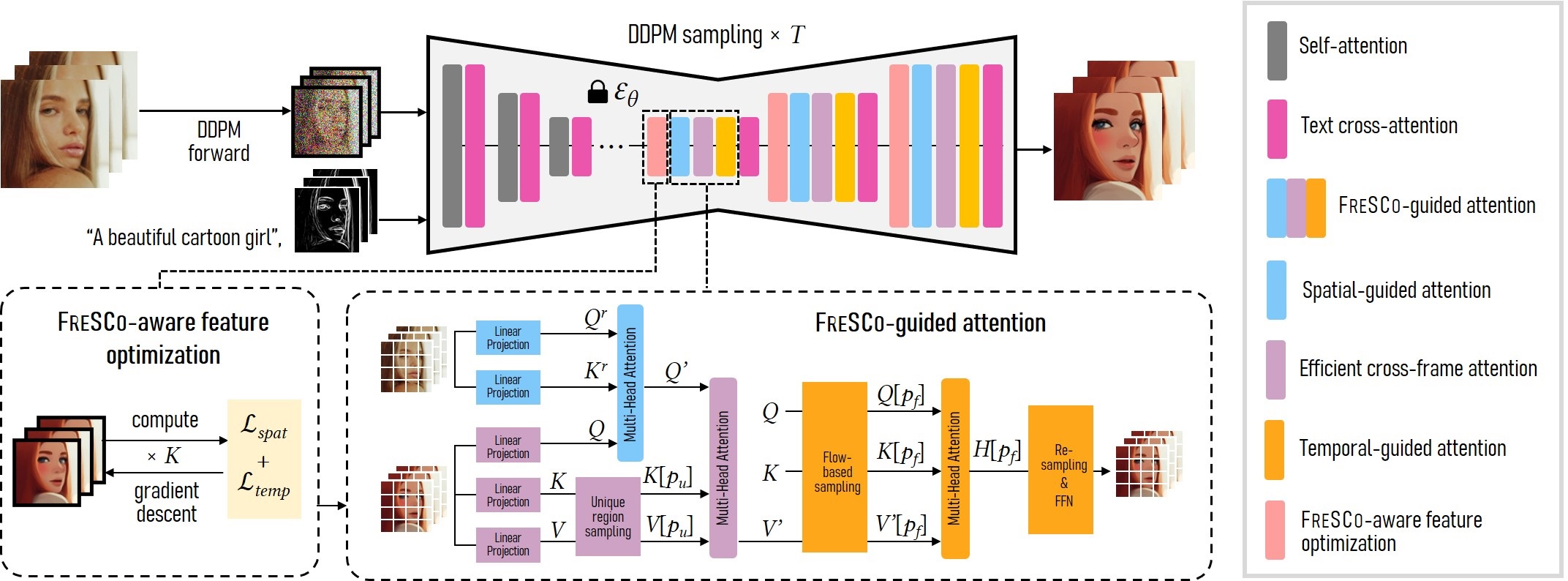
Effect of
Spatial-Temporal Constraints
Our adaptation focuses on incorporating the spatial and temporal correspondences of the input frames I into the U-Net. More specifically, we define temporal and spatial correspondences of I as:
- Temporal correspondence: This inter-frame correspondence is measured by optical flows between adjacent frames, a pivotal element in keeping temporal consistency. Our objective is to ensure that videos share the same optical flow in non-occluded regions before and after manipulation.
- Spatial correspondence: This intra-frame correspondence is gauged by self-similarity among pixels within a single frame. The aim is for the manipulated frame to share self-similarity as the original frame, i.e., semantically similar content is transformed into a similar appearance, and vice versa.
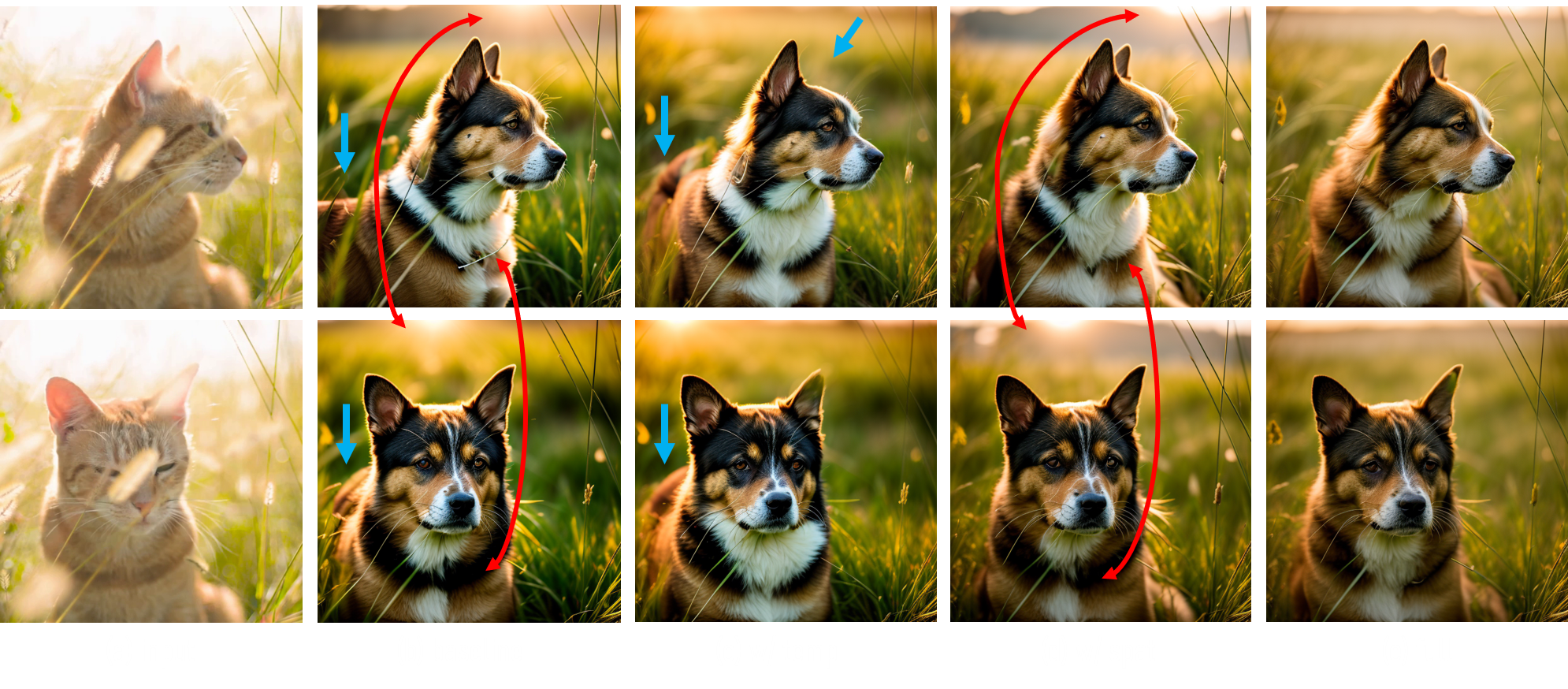
Effect of incorporating spatial and temporal correspondences. The baseline method solely uses cross-frame attention for temporal consistency. By introducing the temporal-related adaptation, we observe improvements in consistency, such as the alignment of textures and the stabilization of the sun's position across two frames. Meanwhile, the spatial-related adaptation aids in preserving the pose during translation. The blue arrows indicate the spatial inconsistency with the input frames. The red arrows indicate the temporal inconsistency between two output frames. Prompt: A dog in the grass in the sun.
Our adaptation focuses on the input feature and the attention module of the decoder layer within the U-Net:
- Feature adaptation: We propose a novel FRESCO-aware feature optimization approach. We design a spatial consistency loss and a temporal consistency loss to directly optimize the decoder-layer features to strengthen their temporal and spatial coherence with the input frames.
- Attention adaptation: We replace self-attentions with FRESCO-guided attentions, comprising three components. Spatial-guided attention first aggregates features based on the self-similarity of the input frame. Then, cross-frame attention is used to aggregate features across all frames. Finally, temporal-guided attention aggregates features along the same optical flow to further reinforce temporal consistency.
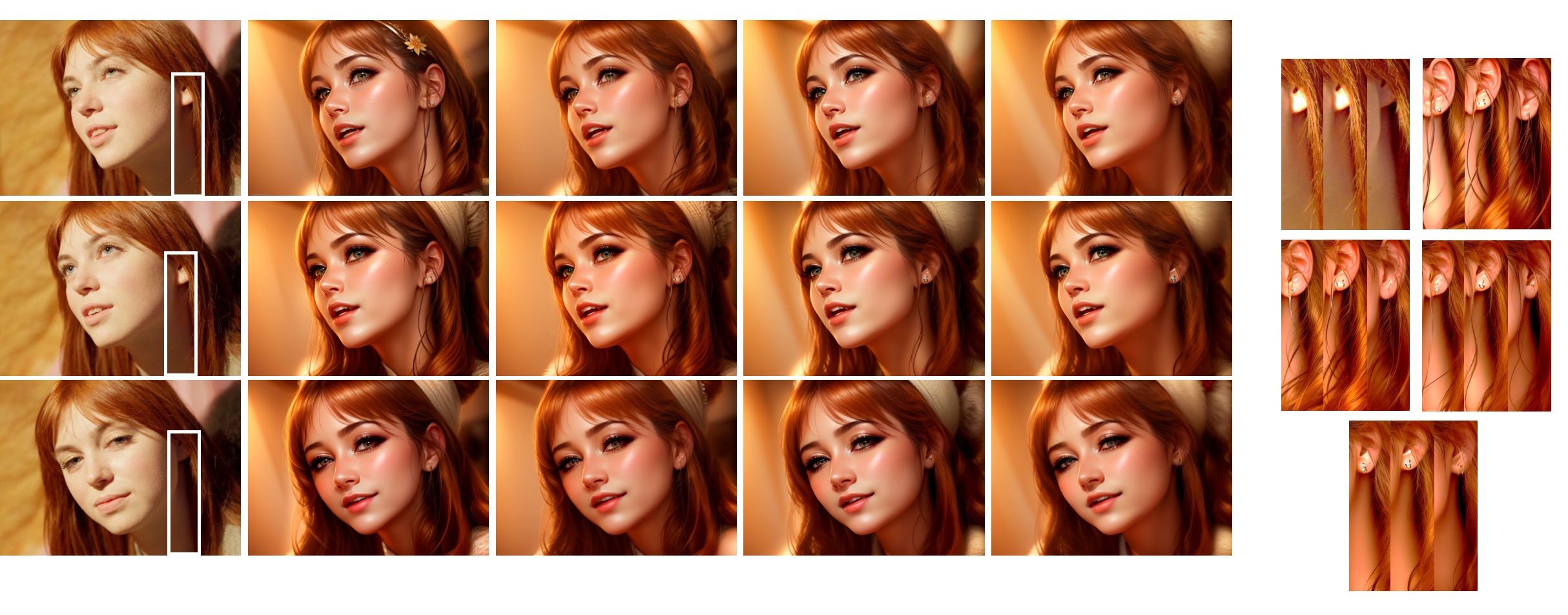
Effect of attention adaptation and feature adaption. Each enhancement individually improves temporal consistency to a certain extent, but neither achieves perfection. Only the combination of the two completely eliminates the inconsistency observed in hair strands. The white region is enlarged with its contrast enhanced on the right for better comparison. Prompt: A beautiful woman in CG style.
Experimental
Results
Comparison with zero-shot text-guided video translation methods
We compare with four recent zero-shot methods: Text2Video-Zero, Rerender-A-Video, ControlVideo, and TokenFlow-pnp. The inversion-based TokenFlow-pnp overfits the input video and somtimes fails to edit the video. Meanwhile, the inversion-free methods, relying on ControlNet conditions, may experience a decline in video editing quality if the conditions are of low quality, due to issues like defocus or motion blur. For instance, ControlVideo fails to generate a plausible appearance of the dog and the boxer. Text2Video-Zero and Rerender-A-Video struggle to maintain the cat's pose and the structure of the boxer's gloves. In contrast, our method can generate consistent videos based on the proposed robust FRESCO guidance.
More results
Paper
Citation
@InProceedings{yang2024fresco,
title = {FRESCO: Spatial-Temporal Correspondence for Zero-Shot Video Translation},
author = {Yang, Shuai and Zhou, Yifan and Liu, Ziwei and and Loy, Chen Change},
booktitle = {Proceedings of IEEE Conference on Computer Vision and Pattern Recognition},
year = {2024},
}
Related
Projects
-
Rerender A Video: Zero-Shot Text-Guided Video-to-Video Translation
S. Yang, Y. Zhou, Z. Liu, C. C. Loy
in ACM SIGGRAPH Asia Conference Proceedings, 2023 (SIGGRAPH Asia)
[PDF] [arXiv] [Project Page] -
StyleGANEX: StyleGAN-Based Manipulation Beyond Cropped Aligned Faces
S. Yang, L. Jiang, Z. Liu, C. C. Loy
in Proceedings of IEEE International Conference on Computer Vision, 2023 (ICCV)
[PDF] [arXiv] [Project Page] -
VToonify: Controllable High-Resolution Portrait Video Style Transfer
S. Yang, L. Jiang, Z. Liu, C. C. Loy
ACM Transactions on Graphics, 2022 (SIGGRAPH Asia - TOG)
[PDF] [arXiv] [Project Page] -
Pastiche Master: Exemplar-Based High-Resolution Portrait Style Transfer
S. Yang, L. Jiang, Z. Liu, C. C. Loy
in Proceedings of IEEE Conference on Computer Vision and Pattern Recognition, 2022 (CVPR)
[PDF] [arXiv] [Project Page]
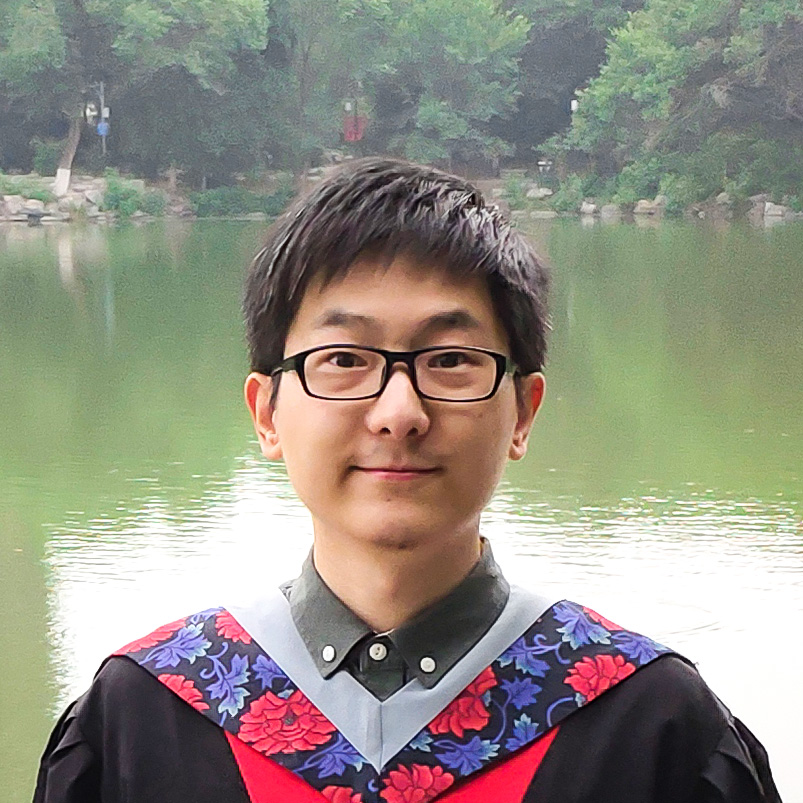